A distance-type measure approach to the analysis of copy number variation in DNA sequencing data
- PMID: 30967117
- PMCID: PMC6456939
- DOI: 10.1186/s12864-019-5491-x
A distance-type measure approach to the analysis of copy number variation in DNA sequencing data
Abstract
Background: The next generation sequencing technology allows us to obtain a large amount of short DNA sequence (DNA-seq) reads at a genome-wide level. DNA-seq data have been increasingly collected during the recent years. Count-type data analysis is a widely used approach for DNA-seq data. However, the related data pre-processing is based on the moving window method, in which a window size need to be defined in order to obtain count-type data. Furthermore, useful information can be reduced after data pre-processing for count-type data.
Results: In this study, we propose to analyze DNA-seq data based on the related distance-type measure. Distances are measured in base pairs (bps) between two adjacent alignments of short reads mapped to a reference genome. Our experimental data based simulation study confirms the advantages of distance-type measure approach in both detection power and detection accuracy. Furthermore, we propose artificial censoring for the distance data so that distances larger than a given value are considered potential outliers. Our purpose is to simplify the pre-processing of DNA-seq data. Statistically, we consider a mixture of right censored geometric distributions to model the distance data. Additionally, to reduce the GC-content bias, we extend the mixture model to a mixture of generalized linear models (GLMs). The estimation of model can be achieved by the Newton-Raphson algorithm as well as the Expectation-Maximization (E-M) algorithm. We have conducted simulations to evaluate the performance of our approach. Based on the rank based inverse normal transformation of distance data, we can obtain the related z-values for a follow-up analysis. For an illustration, an application to the DNA-seq data from a pair of normal and tumor cell lines is presented with a change-point analysis of z-values to detect DNA copy number alterations.
Conclusion: Our distance-type measure approach is novel. It does not require either a fixed or a sliding window procedure for generating count-type data. Its advantages have been demonstrated by our simulation studies and its practical usefulness has been illustrated by an experimental data application.
Keywords: Copy number variation; DNA; Distance-type measure; Genome-wide sequencing; Geometric distribution; Mixture model.
Conflict of interest statement
Ethics approval and consent to participate
Not applicable.
Consent for publication
The authors agree the consent for publication.
Competing interests
The authors declare that they have no competing interests.
Publisher’s Note
Springer Nature remains neutral with regard to jurisdictional claims in published maps and institutional affiliations.
Figures
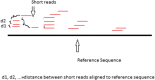
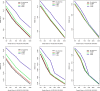
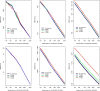
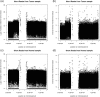
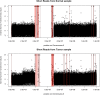
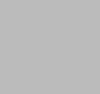
Similar articles
-
PSE-HMM: genome-wide CNV detection from NGS data using an HMM with Position-Specific Emission probabilities.BMC Bioinformatics. 2016 Nov 3;18(1):30. doi: 10.1186/s12859-016-1296-y. BMC Bioinformatics. 2016. PMID: 27809781 Free PMC article.
-
SCOPE: A Normalization and Copy-Number Estimation Method for Single-Cell DNA Sequencing.Cell Syst. 2020 May 20;10(5):445-452.e6. doi: 10.1016/j.cels.2020.03.005. Cell Syst. 2020. PMID: 32437686 Free PMC article.
-
rSW-seq: algorithm for detection of copy number alterations in deep sequencing data.BMC Bioinformatics. 2010 Aug 18;11:432. doi: 10.1186/1471-2105-11-432. BMC Bioinformatics. 2010. PMID: 20718989 Free PMC article.
-
Genome structural variation discovery and genotyping.Nat Rev Genet. 2011 May;12(5):363-76. doi: 10.1038/nrg2958. Epub 2011 Mar 1. Nat Rev Genet. 2011. PMID: 21358748 Free PMC article. Review.
-
Beyond assembly: the increasing flexibility of single-molecule sequencing technology.Nat Rev Genet. 2023 Sep;24(9):627-641. doi: 10.1038/s41576-023-00600-1. Epub 2023 May 9. Nat Rev Genet. 2023. PMID: 37161088 Free PMC article. Review.
References
MeSH terms
LinkOut - more resources
Full Text Sources
Miscellaneous