Leveraging FAM features to predict the prognosis of LGG patients and immunotherapy outcome
- PMID: 39005680
- PMCID: PMC11236777
- DOI: 10.62347/GIGO3446
Leveraging FAM features to predict the prognosis of LGG patients and immunotherapy outcome
Abstract
Heterogeneity at biological and transcriptomic levels poses a challenge in defining and typing low-grade glioma (LGG), leading to a critical need for specific molecular signatures to enhance diagnosis, therapy, and prognostic evaluation of LGG. This study focused on fatty acid metabolism (FAM) related genes and prognostic features to investigate the mechanisms and treatment strategies for LGG cell metastasis and invasion. By screening 158 FAM-related genes and clustering 512 LGG samples into two subtypes (C1 and C2), differential gene expression analysis and functional enrichment were performed. The immune cell scores and prognosis were compared between the two subtypes, with C1 showing poorer outcomes and higher immune scores. A four-gene signature (PHEX, SHANK2, HOPX, and LGALS1) was identified and validated across different datasets, demonstrating a stable predictive effect. Cellular experiments confirmed the roles of LGALS1 and HOPX in promoting tumor cell proliferation, migration, and invasion, while SHANK2 exhibited a suppressive effect. This four-gene signature based on FAM-related genes offers valuable insights for understanding the pathogenesis and clinical management of LGG.
Keywords: HOPX; LGG; PHEX; SHANK2; Tumor immunology; and LGALS1; fatty acid metabolism; prognostic features.
AJCR Copyright © 2024.
Conflict of interest statement
None.
Figures
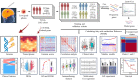
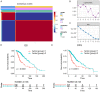
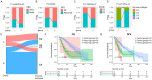
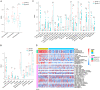
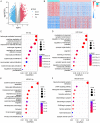
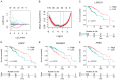
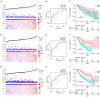
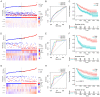
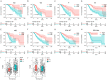
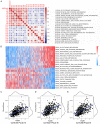
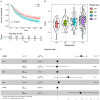
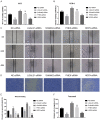
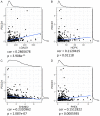
Similar articles
-
Identification and clinical validation of diverse cell-death patterns-associated prognostic features among low-grade gliomas.Sci Rep. 2024 May 24;14(1):11874. doi: 10.1038/s41598-024-62869-4. Sci Rep. 2024. PMID: 38789729 Free PMC article.
-
Integrated analysis of inflammatory response subtype-related signature to predict clinical outcomes, immune status and drug targets in lower-grade glioma.Front Pharmacol. 2022 Aug 26;13:914667. doi: 10.3389/fphar.2022.914667. eCollection 2022. Front Pharmacol. 2022. PMID: 36091778 Free PMC article.
-
Construction of a novel molecular typing and scoring system for anoikis distinguishes between different prognostic risks and treatment responsiveness in low-grade glioma.Front Immunol. 2023 Apr 11;14:1105210. doi: 10.3389/fimmu.2023.1105210. eCollection 2023. Front Immunol. 2023. PMID: 37114037 Free PMC article.
-
Machine learning identification of cuproptosis and necroptosis-associated molecular subtypes to aid in prognosis assessment and immunotherapy response prediction in low-grade glioma.Front Genet. 2022 Sep 12;13:951239. doi: 10.3389/fgene.2022.951239. eCollection 2022. Front Genet. 2022. PMID: 36186436 Free PMC article.
-
Identification of a novel cuproptosis-related gene signature and integrative analyses in patients with lower-grade gliomas.Front Immunol. 2022 Aug 15;13:933973. doi: 10.3389/fimmu.2022.933973. eCollection 2022. Front Immunol. 2022. PMID: 36045691 Free PMC article. Review.
References
-
- Reifenberger G, Wirsching HG, Knobbe-Thomsen CB, Weller M. Advances in the molecular genetics of gliomas - implications for classification and therapy. Nat Rev Clin Oncol. 2017;14:434–452. - PubMed
-
- Horbinski C, Berger T, Packer RJ, Wen PY. Clinical implications of the 2021 edition of the WHO classification of central nervous system tumours. Nat Rev Neurol. 2022;18:515–529. - PubMed
LinkOut - more resources
Full Text Sources
Research Materials
Miscellaneous